Keynote speakers
Daniel Creemers (Professor, Technische Universität München) |
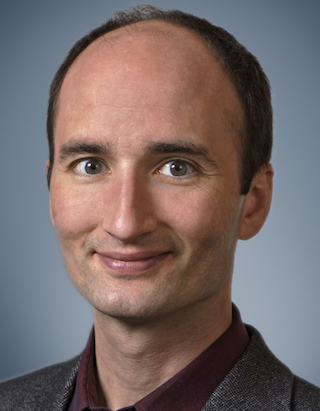 |
Biography: Daniel Cremers received Bachelor degrees in Mathematics (1994) and Physics (1994), and a Master's degree in Theoretical Physics (1997) from the University of Heidelberg. In 2002 he obtained a PhD in Computer Science from the University of Mannheim, Germany. Subsequently he spent two years as a postdoctoral researcher at the University of California at Los Angeles (UCLA) and one year as a permanent researcher at Siemens Corporate Research in Princeton, NJ. From 2005 until 2009 he was associate professor at the University of Bonn, Germany. Since 2009 he holds the Chair of Computer Vision and Artificial Intelligence at the Technical University of Munich. His publications received several awards, including the 'Best Paper of the Year 2003' (Int. Pattern Recognition Society), the 'Olympus Award 2004' (German Soc. for Pattern Recognition) and the '2005 UCLA Chancellor's Award for Postdoctoral Research'. For pioneering research he received a Starting Grant (2009), two Proof of Concept Grants (2014 & 2018), a Consolidator Grant (2015) and an Advanced Grant (2020) by the European Research Council. Professor Cremers has served as associate editor for several journals including the International Journal of Computer Vision, the IEEE Transactions on Pattern Analysis and Machine Intelligence and the SIAM Journal of Imaging Sciences. He has served as area chair (associate editor) for ICCV, ECCV, CVPR, ACCV, IROS, etc, and as program chair for ACCV 2014. In 2018 he organized the largest ever European Conference on Computer Vision in Munich with 3300 delegates. He is member of the Bavarian Academy of Sciences and Humanities. He is honorary member of the Dagstuhl Scientific Directorate. In December 2010 he was listed among "Germany's top 40 researchers below 40" (Capital). On March 1st 2016, Prof. Cremers received the Gottfried Wilhelm Leibniz Award, the biggest award in German academia. According to Google Scholar, Prof. Cremers has an h-index of 92 and his papers have been cited 39198 times. According to Guide2Research he is among the most influential scientists in Germany. He is co-founder of several companies, most recently the high-tech startup Artisense.
Title: Visual SLAM in the Age of Deep Learning
Abstract : While neural networks have swept the field of computer vision and replaced classical methods in most areas of image analysis and beyond, extending their power to the domain of camera-based 3D reconstruction and visual SLAM remains an important challenge. In my talk, I will discuss the problem of image-based reconstruction and visual Simultaneous Localization and Mapping (SLAM). In particular, I will advocate direct methods that recover 3D structure and camera motion directly from the intensity images. Moreover, I will discuss how the performance of visual SLAM methods can be drastically enhanced using the predictive power of deep networks trained in a self-supervised manner.
Video: here
|
Julie Delon (Professor at MAP5, Université Paris Descartes)
|
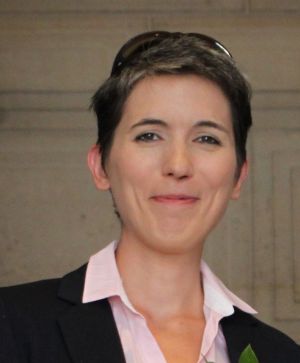 |
Biography: Julie Delon is full professor of mathematics at Université de Paris. She is a member of the MAP5 laboratory, UMR 8145, and she has been elected a member of the Institut universitaire de France. She is an associate editor for Image Processing on Line. Her research interest includes stochastic and statistical modeling for image editing and restoration, and numerical optimal transport for imaging and computer vision. In 2018, she received the Blaise Pascal award from the French Academy of Sciences.
Title: Some perspectives on stochastic models for Bayesian image restoration
Abstract: Random image models are central for solving inverse problems in imaging. In a Bayesian formalism, these models can be used as priors or regularisers and combined to an explicit likelihood function to define posterior distributions. Most of the time, these posterior distributions are used to derive Maximum A Posteriori (MAP) estimators, leading to optimization problems that may be convex or not, but are well studied and understood. Sampling schemes can also be used to explore these posterior distributions, to derive Minimum Mean Square Error (MMSE) estimators, quantify uncertainty or perform other advanced inferences. While research on inverse problems has focused for many years on explicit image models (either directly in the image space, or in a transformed space), an important trend nowadays is to use implicit image models encoded by neural networks. This opens the way to restoration algorithms that exploit more powerful and accurate prior models for natural images but raises novel challenges and questions on the corresponding posterior distributions and their resulting estimators. The goal of this presentation is to provide some perspectives and present recent developments on these questions.
|
Carola-Bibiane Schönlieb (Professor and head of the Cambridge Image Analysis, University of Cambridge)
|
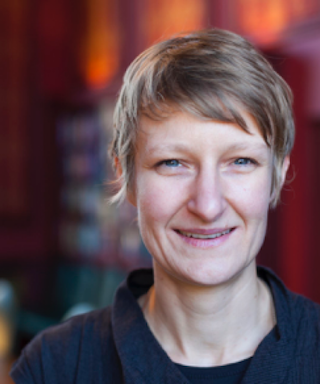 |
Biography: Carola-Bibiane Schönlieb is a Professor of Applied Mathematics and head of the Cambridge Image Analysis (CIA) group at the Department of Applied Mathematics and Theoretical Physics (DAMTP), University of Cambridge. She is also the Director of the Cantab Capital Institute for the Mathematics of Information, Director of the EPSRC Centre for Mathematical and Statistical Analysis of Multimodal Clinical Imaging, a Fellow of Jesus College, Cambridge and co-leader of the IMAGES network.
Title: Machine Learned Regularisation for Inverse Imaging Problems
Abstract: Inverse problems are about the reconstruction of an unknown physical quantity from indirect measurements. In imaging, they appear in a variety of places, from medical imaging, for instance MRI or CT, to remote sensing, for instance Radar, to material sciences and molecular biology, for instance electron microscopy. Here, imaging is a tool for looking inside specimen, resolving structures beyond the scale visible to the naked eye, and to quantify them. It is a mean for diagnosis, prediction and discovery.
Most inverse problems of interest are ill-posed and require appropriate mathematical treatment for recovering meaningful solutions. Regularization is one of the main mechanisms to turn inverse problems into well-posed ones by adding prior information about the unknown quantity to the problem, often in the form of assumed regularity of solutions.
Classically, such regularization approaches are handcrafted. Examples include Tikhonov regularization, the total variation and several sparsity-promoting regularizers such as the L1 norm of Wavelet coefficients of the solution. While such handcrafted approaches deliver mathematically and computationally robust solutions to inverse problems, providing a universal approach to their solution, they are also limited by our ability to model solution properties and to realise these regularization approaches computationally.
Recently, a new paradigm has been introduced to the regularization of inverse problems, which derives regularization approaches for inverse problems in a data driven way. Here, regularization is not mathematically modelled in the classical sense, but modelled by highly over-parametrised models, typically deep neural networks, that are adapted to the inverse problems at hand by appropriately selected (and usually plenty of) training data.
In this talk, I will review some machine learning based regularization techniques, present some work on unsupervised and deeply learned convex regularisers and their application to image reconstruction from tomographic and blurred measurements, and finish by discussing some open mathematical problems.
Video (partial): here
|
Jean-Luc Starck (Director of CosmoStat, CEA)
|
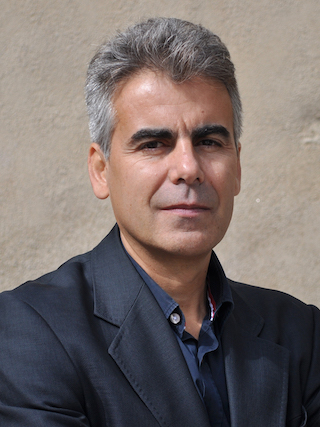 |
Biography: Jean-Luc Starck is Director of Research and head of the CosmoStat laboratory at the Institute of Research into the Fundamental Laws of the Universe, Département d'Astrophysique, CEA-Saclay, France. Jean-Luc Starck has a Ph.D from Nice Observatory and an Habilitation from University Paris XI. He was a visitor at the European Southern Observatory in 1993, at UCLA in 2004 and at Stanford’s Department of Statistics in 2000 and 2005. Since 1994, he is a tenured researcher at CEA. He created in 2010 the CosmoStat laboratory and is strongly involved in the Euclid ESA space mission. He received the EADS prize of the French Academy of Science in 2011, the International Astrostatistics Association (IAA) Fellow Prize in 2016 and the 2018 Gruber Price in Cosmology (as member of the ESA Planck team). He leads Cosmostat, an interdisciplinary research group at the interface between cosmology and statistical methods with a focus on industry-academia partnership. He has organized 16 conferences, and was keynote, invited or seminar speaker over fifty times in the last five years. Over the last 10 years, he has been involved as Co-I or PI in the management of 8 million euros of grants from national, European and international sources, including a senior ERC. He has published over 200 refereed papers in astrophysics, cosmology, signal processing and applied mathematics, and he is also author of three books.
Title: Bridging the gap between U-nets and sparse recovery
Abstract: U-net neural networks have provided very impressive results and are now considered as state of art techniques for many inverse problems. But we do not necessarily understand the reasons which make U-nets so powerful. Sparsity techniques have dominated for many years the field, but are clearly less efficient than U-net. But contrary to U-nets, sparsity is well understood and present theoretical guarantees which make sparse recovery still an interesting approach, especially for medical applications. Many papers have underlined similarities between U-nets and sparse recovery. Here, we present a new approach, the learnlet transform, which belongs to the family of sparse decompositions, but using all ingredients of neural networks. We show through denoising experiments that learnlet outperforms other sparse denoising techniques, being however less efficient that U-net in term of MSE. Learnlet denoising presents very interesting properties such as a much smaller number of parameters to learn, a full understanding of how it works and a very important, a better generalization than U-net when the noise in the data is larger than the noise level used in the training.
|
|